What does a starfish, some thorns from a tangerine tree, chicken eggs and AI have to do with each other? Let me introduce you to the language of cells and how we learn it with the help of AI.
I have found books on the latest developments in biomedicine and immunology very insightful. An example would be the work of Matt Richtel: “An Elegant Defense” (see here). I am currently reading the fascinating book from Siddhartha Mukherjee: “The Song of the Cell” (highly recommend it), which happens to touch on the work of Ilya Mechnikov and Stanley Cohen and the discoveries of Phagocytes and Cytokines.
“Essentially, cytokines are the vocabulary of the language that cells use to communicate with one another” – says Stanley Cohen. Cytokines – “cyto to represent “cell,” and kine, because it reminded Cohen of the action word “kinetic,” and because, well, it sounded nice after cyto. In science, Stan says, “nothing seems real until you name it.”“1
Recent research developments have shown how complex the signaling system immune cells use to coordinate responses to infection and cancer actually is. It is comparable to deciphering a new language. This sounds oddly familiar to training AI with NLP…
After training AI on how we communicate through language as humans, it is a fascinating idea to try to better understand how the vocabulary of cells work and how immune cells communicate. Wouldn’t it be great to apply some of the new developments in AI and advancements in GenAI capability to the vocabulary of cells? In her article: “Applying AI to Immune Cell Networks” Rachel Thomas provides a fantastic example of the real impact of understanding AI and applying it to innovation in areas, such as biomedicine.
From Starfish to Chicken Eggs
Rachel Thomas references the importance of better understanding cell communication: “Immune cell communication through cytokines is a key area for us to better understand medicine and disease. Various immune cell types must communicate to coordinate their response to threats. However, the immune system may end up over-reacting, under-reacting, or having a misplaced reaction, all of which can cause disease. Sepsis occurs when the immune system responds too vigorously, damaging our own organs. In cancer, the immune system may under-respond, failing to attack cancerous cells that it should. Several types of cancer therapies involve trying to activate or reactivate our own immune cells. In other cases, the immune system mistakenly attacks our own tissue, causing autoimmune diseases including Type 1 diabetes, rheumatoid arthritis, multiple sclerosis, and psoriasis.“2 You can read part 1 of her 2 part series here.
Reading “The Song of the Cell” got me interested to dive a little bit deeper into how we got to know the vocabulary the immune system uses to communicate. The history of the main discoveries of immunology is full of fascinating stories. In this blog I wanted to follow the work of 2 scientists, which lead to the discovery of Cytokines.
Born 1845 in a village near Kharkiv (Ukraine), the story of Ilya Mechnikov is ripe for a movie adoption in itself. Having survived 2 suicide attempts (one from overdosing on opium and one from inoculating himself with relapsing fever), his ground breaking discovery involved stabbing starfish larvae with tangerine tree needles from his family’s Christmas tree. Mechnikov hypothesised that the new cells surrounding the needles overnight might take up and digest bacteria that get into the body: he named them Phagocytes. This discovery seemed to also have saved his life: “he abandoned his pessimistic philosophy and determined to find further proof of his hypothesis.“3 In 1908 he shared the Nobel Prize in Physiology or Medicine for his discovery together with Paul Ehrlich.
Mechnikov noted that the immune cells were attracted to the site of inflammation autonomously, “thanks to a sort of spontaneous action“. It would take till 1974 for a specific type of protein to be “discovered” by Stanley Cohen triggering this action as part of cell signaling.
Cohen was researching lymphocytes, then the newly discovered source of the antibodies fighting viruses and other invaders. He made his discovery thanks to a failed experiment to grow enough virus to proof that viral infection can lead to immune suppression in chicken eggs. Given the eggs had no immune system, he was surprised to find that they did in fact have an immune response. His experiments led to the hypothesis “that most if not all cells could secrete factors that affected the behavior of other cells (not just lymphocytes), and that the phenomenon went beyond just the immune system.“4
Cytokines are a byproduct of the failed experiment and a great reminder “that the most interesting things sometimes come out of results that are negative. Why discard something without seeing what’s going on?”5
immuneXpresso
One of the major problems in an area of research focused on cytokines, since the discovery of Cohen? The research field (and it’s data) exploded!
“Knowledge of the immune intercellular network is crucial for understanding immune responses in health and disease. However, the high system complexity leaves even expert researchers struggling to maintain a mental picture of the immune milieu and often leads to knowledge biases.“6
In 2018 a group of researches (Kveler, Ksenya, Elina Starosvetsky, Amit Ziv-Kenet, Yuval Kalugny, Yuri Gorelik, Gali Shalev-Malul, Netta Aizenbud-Reshef et al.) tried to bring order to the chaos of publications and built immuneXpresso: a database of relevant articles in this developing area of research. Keep in mind that their work included a total of 16(!) authors.
Their paper “Immune-centric network of cytokines and cells in disease context identified by computational mining of PubMed” shows the challenges of this research in the fantastic 2 images below.
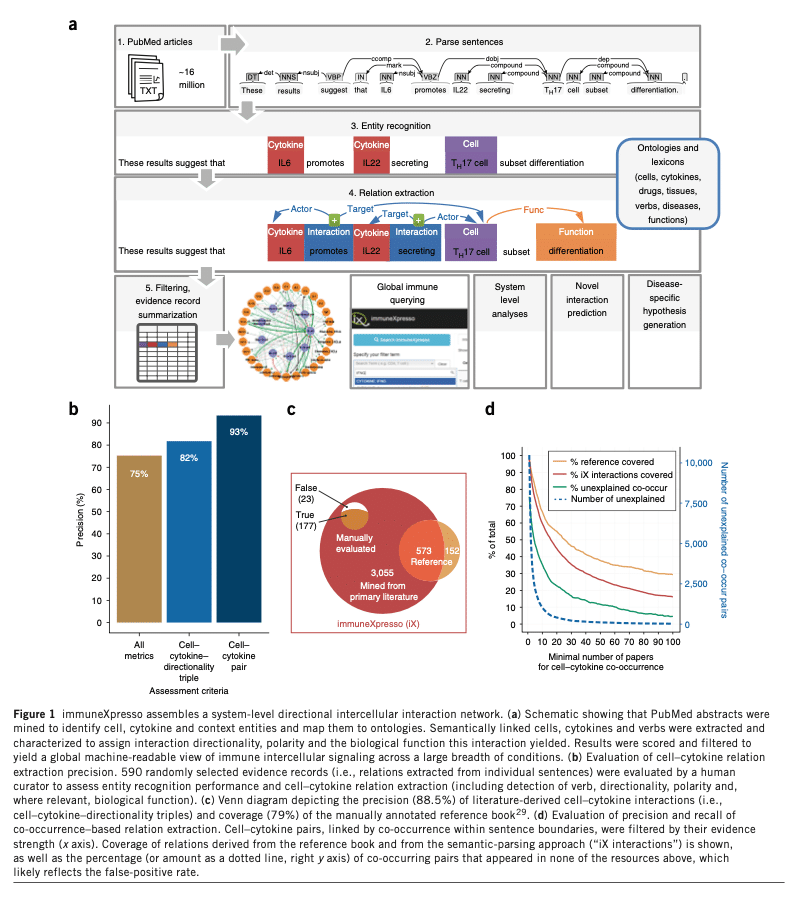
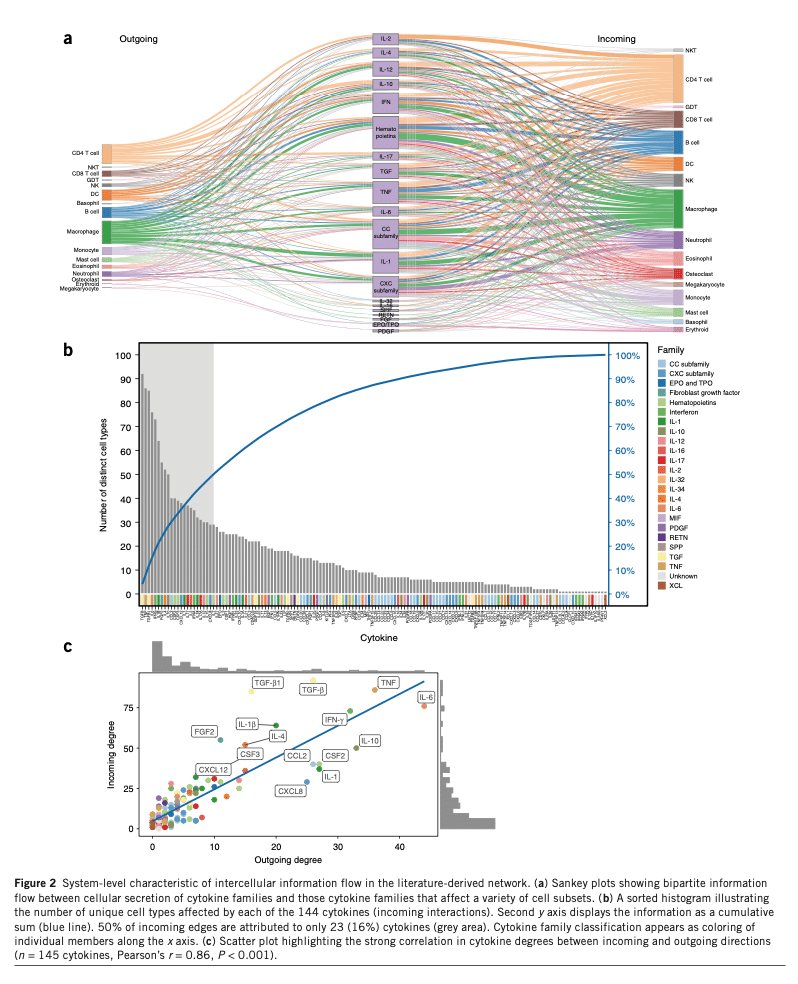
The researchers predicted possible cytokine-diseases associations have not been identified due to the massive influx of research and complexity in the data. Their finding showed that 2 examples of the impact of the chemokines CCL8 and CCL24 in psoriasis have not been reported.
Their conclusion hints at what has emerged as an area of applying AI and ML algorithms since: “The extensive metadata we extracted for each article, including MESH terms and bibliographic information, together with detailed characterization of the captured interactions, could be used for advanced filtering, which would allow focus on the most authoritative knowledge. Beyond this, we envision that the structured formatting of knowledge we have achieved can be leveraged by machine-learning applications, using statistical analyses of domain frequency and chronological pattern biases to identify potential discrepancies and erroneous claims in the published knowledge.“
Let’s have a look at some of the new developments since 2018.
From Network Expansion to Transformers
The latest improvement of immuneXpresso I could find in research literature is ENQUIRE (Expanding Networks by Querying Unexpectedly Inter-Related Entities). It references other developments since 2018, but again shows the importance of better understanding the latest research literature.
The paper by Luca Musella, Max Widmann and Julio Vera emphasises the importance of relevancy of the PubMed sources: “ENQUIRE processes scientific articles by recognizing gene mentions in abstracts and extracting Medical Subject Headings (MeSH) to enrich gene-gene co-occurrences with contextual information. It applies state-of-the-art methods in statistical network analysis to reconstruct a network from an input corpus and generate relevant PubMed queries, to contextually expand the underlying corpus and, in turn, the network. A distinctive element in our methodology is the use of a statistical framework that accounts for literature biases. In this study, we present the tool, discuss the features of its underlying algorithm, and assess ENQUIRE’s applicability and effectiveness with three concrete test cases.“7
Another example of the impact of AI developments is the application of Transformers. Tang W, at al. in their paper “Single-Cell Multimodal Prediction via Transformers“8 developed scMoFormer via the application of a multimodal transformer to improve the application of graph neural networks. The result is promising: “Remarkably, scMoFormer achieves superior and more stable performance than other baselines on both 2021 and 2022 NeurIPS single-cell datasets.“9
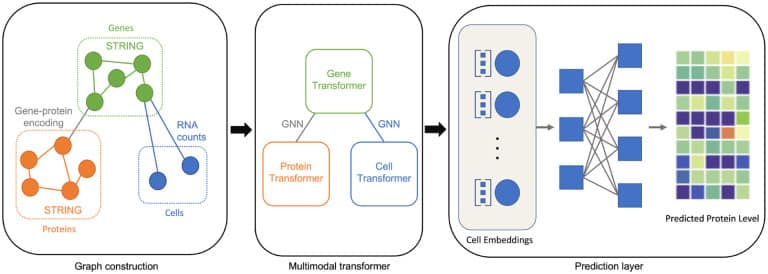
An illustration of scMoFormer. In this framework, three important components are included: graph construction, multimodal transformer, and prediction layer.
Then there is the release of CINEMA-OT (Causal independent effect module attribution + optimal transport)10 – a software to help examine a cell’s response to different cytokine combinations. Below are 2 interesting overviews from the study:
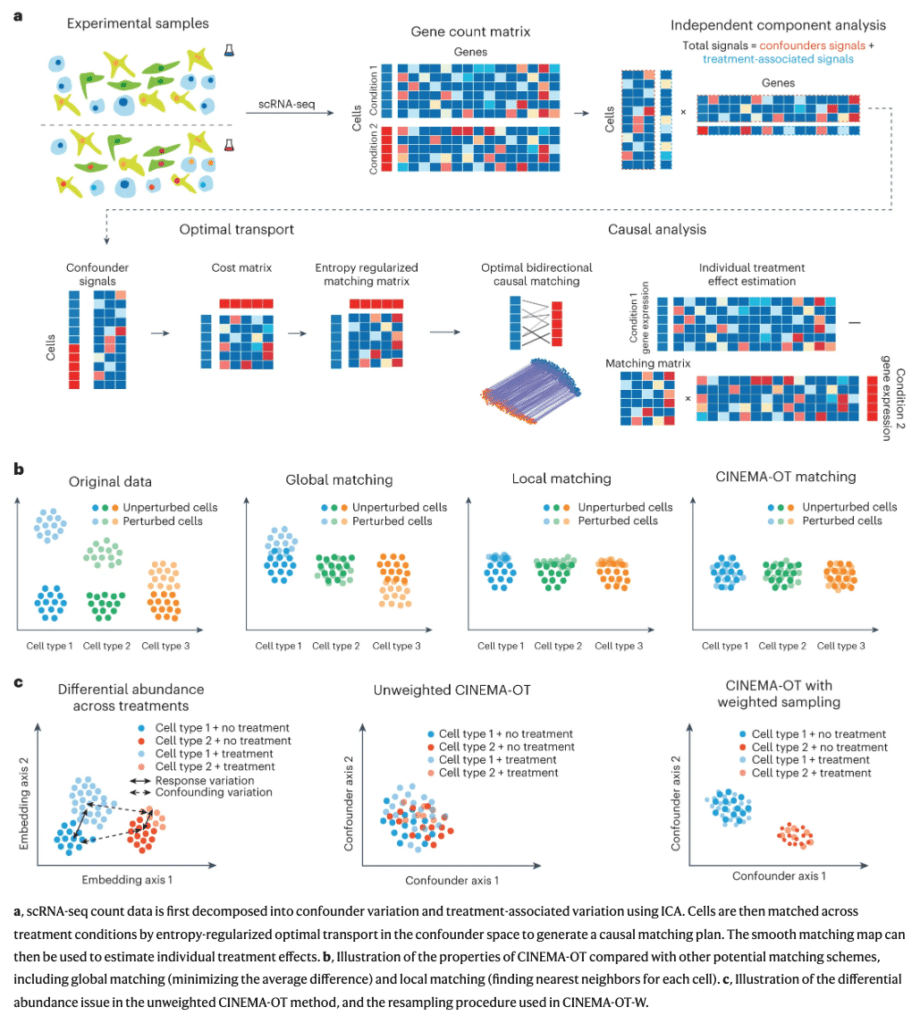
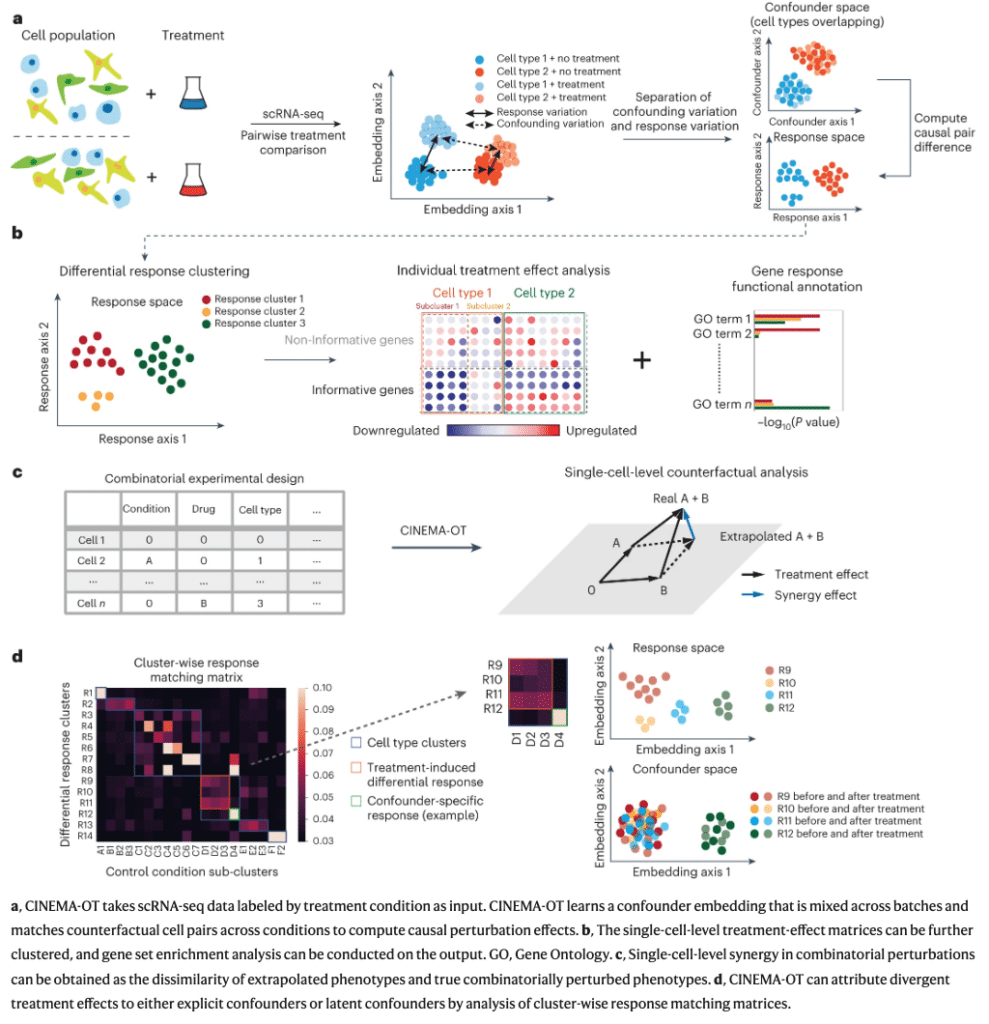
ENQUIRE, scMoFormer and CINEMA-OT are just some of the specialised tools I was able to find in my brief research. The real impact of the accelerating speed of AI developments, especially in the open source space have brought up another question though: can you apply an understanding of general AI research to specialised field of biomedicine? Or in the case of Jeff Hammerbach and his colleagues: Can you transfer what you have learned at Facebook to cancer research?
I love Thomas’ references of the work of Czech and Hammerbach in their paper: “Extracting T Cell Function and Differentiation Characteristics from the Biomedical Literature“11 and encourage you to listen to the talk Jeff Hammerbacher gives here.
So can you transfer what you have learned at Facebook to cancer research? It turns out that you can…
Learning a new language
One of the very first applications of NLP in ML is the application of tokenization. An area becoming quite important in medicine research as well when comparing “Th1 (CD4+IL-17-IFN-γhi) cells” with “helper CD4+IL-17-IFN-γhi type 1 cells” and trying to identify that these are synonymous examples of the same thing. Oh and keep the Th1 cell and CD4 in mind for later. We are going to look it up and see how it actually looks.
A huge challenge of advancing biomedicine research is to identify current papers on state-of-the-art research when you learn the language of cells. Especially as a growing number of researchers around the globe are driving this cutting edge research to unlock new immune therapies. You might have heard of T cells before, but it is not that easy to understand how various different forms of T cells are created or even identified. Which is where cytokines come in. Jeff Hammerbach gives a perfect example on how Cytokines can be inducing or be secreted. In order to analyse the PubMed documents, they had to leverage existing python libraries for classifying cell names: ScispaCy.
Mark Neumann, Daniel King, Iz Beltagy and Waleed Ammar introduced a new python library in 2019 to help apply better tokenization in biomedicine: “ScispaCy: Fast and Robust Models for Biomedical Natural Language Processing“12 Hammerbach and Czech reviewed the tokenization and found that they had to go back to the drawing board to reset the tokenizer. The outcome in 2019? Biomedicine needed more labeled data to better apply AI/ML and researchers improve their labeling.
“This work also demonstrates that with the help of weak supervision, a single or small number of bioinformatician(s) can develop relation extraction processes with around 20 hours of annotation time expected per relation type, which may represent an improvement over the 16 authors and 11 human annotators that contributed to immuneXpresso.“13
It is in the application of faster and cheaper approaches and models and the growth of data that new opportunities are emerging.
LLMs and Fine-tuning
Scientific breakthroughs made gene sequencing vastly cheaper and hence the data available to train AI models is growing exponentially. Cell data can be tokenized as we have seen and treated as textual data. Large language models (LLMs) can be trained (or fine-tuned) solely on these new forms of input data to develop a nuanced understanding of biology. The idea is that with the new vocabulary being understood by these new AI algorithms, we may be able to not just understand existing cells, but develop entirely new proteins as well.
In 2020 Salesforce Research released “ProGen: Language Modeling for Protein Generation“14. The highly specialized LLM is helping with generating new proteins to help accompany existing state-of-the-art methods in this field.
In 2021 Google’s Deepmind released the paper on AlphaFold: “Highly accurate protein structure prediction with AlphaFold“15. Below is an overview of how AlphaFold works:
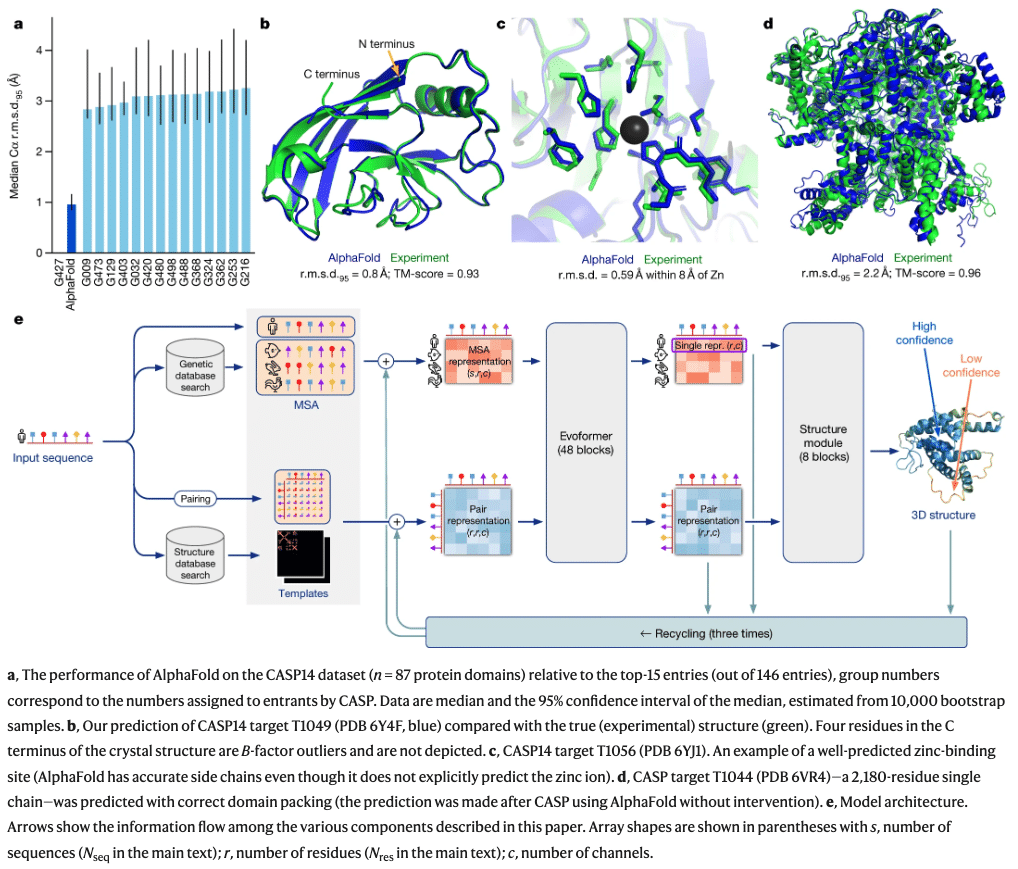
The goal of AlphaFold is to keep up with the explosion of genomic data: “The explosion in available genomic sequencing techniques and data has revolutionized bioinformatics but the intrinsic challenge of experimental structure determination has prevented a similar expansion in our structural knowledge. By developing an accurate protein structure prediction algorithm, coupled with existing large and well-curated structure and sequence databases assembled by the experimental community, we hope to accelerate the advancement of structural bioinformatics that can keep pace with the genomics revolution.“16
The great thing about AlphaFold is that you can look up protein structures. Remember the T-cell Th1 CD+ cell? Have a look at the: Mutant T-cell surface glycoprotein CD4 here. As a reminder, why this glycoprotein matters: “CD4+ T helper cells are white blood cells that are an essential part of the human immune system. They are often referred to as CD4 cells, T-helper cells or T4 cells. They are called helper cells because one of their main roles is to send signals to other types of immune cells, including CD8 killer cells, which then destroy the infectious particle.“17
The big question will be, if LLMs will play a more important role than fine-tuned models? I guess it will be a balance of both. Fine-tuning can improve the ability to perform a very specialised task, like generating protein sequences with Salesforce’s ProGen. As general LLMs are becoming more and more powerful, it will be interesting to see how they will have impact in this field as well. Given that one of the key challenges of advancing studies in biomedicine is the scanning of PubMed. In 2022 PubMed citations had a total of more than 34M entries and reached more than 2.58 Billion searches.
I hope you found this little exploration of the advancements of biomedicine insightful. I am looking forward to part 2 of Rachel Thomas’ 2 part series and recommend you have a look at “The Song Of The Cell”.
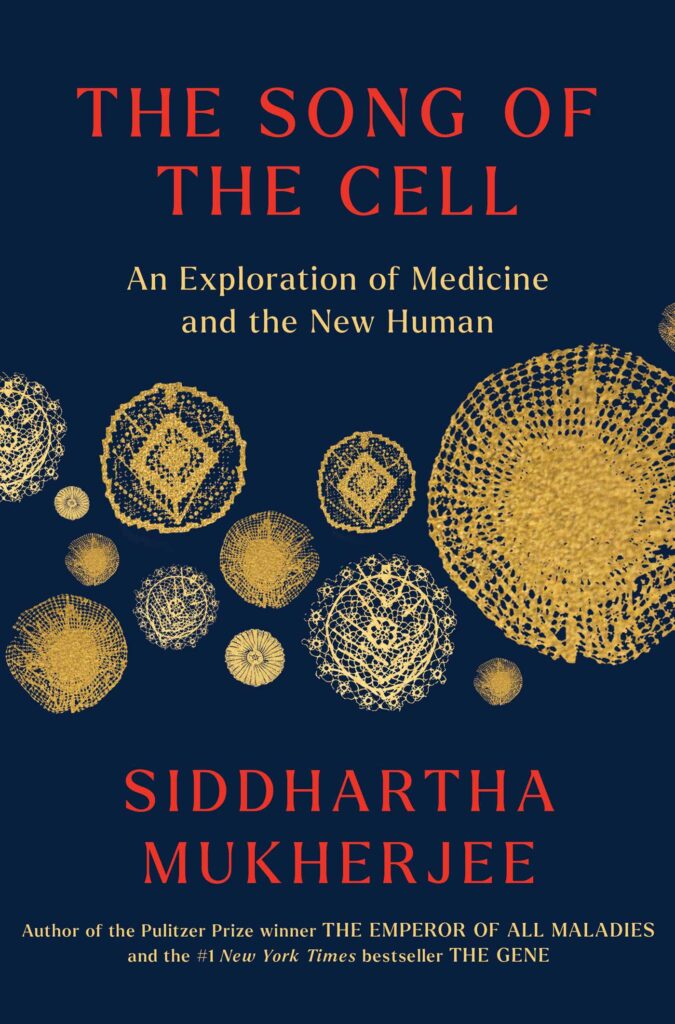
“The Song of the Cell: An Exploration of Medicine and the New Human” by Siddhartha Mukherjee presents a comprehensive journey into cellular biology and its transformative role in modern medicine. Mukherjee, a renowned oncologist and author, explores the cell as life’s fundamental unit, offering deep insights into how cellular understanding has revolutionized medical science. The book delves into the discovery of cells, their complex functions, and their impact on understanding diseases. It highlights key advancements in cellular research and therapy, including the development of targeted cancer treatments and regenerative medicine.
Mukherjee skillfully intertwines scientific exploration with compelling patient stories, showcasing the real-world impact of cellular discoveries on human health. He discusses how newfound knowledge of cells has led to innovative treatments for a variety of illnesses, fundamentally altering medical approaches and patient outcomes. The narrative also contemplates the ethical and philosophical implications of these advancements, particularly in the context of genetic engineering and personalized medicine.
Overall, the book is not only a celebration of scientific achievement but also a thoughtful examination of the challenges and responsibilities that come with newfound medical powers. It serves as an enlightening resource for anyone interested in the intersection of biology, medicine, and technology.
ChatGPT-4’s summary
- 2018: Chickens, Cells and Cytokines ↩︎
- Applying AI to Immune Cell Networks ↩︎
- Ilya Ilyich Mechnikov – The Nobel Prize in Physiology or Medicine 1908 ↩︎
- 2018: Chickens, Cells and Cytokines ↩︎
- 2018: Chickens, Cells and Cytokines ↩︎
- Immune-centric network of cytokines and cells in disease context identified by computational mining of PubMed ↩︎
- ENQUIRE RECONSTRUCTS AND EXPANDS GENE AND MESH CO-OCCURRENCE NETWORKS FROM CONTEXT-SPECIFIC LITERATURE ↩︎
- Single-Cell Multimodal Prediction via Transformers ↩︎
- Single-Cell Multimodal Prediction via Transformers ↩︎
- https://www.nature.com/articles/s41592-023-02040-5 ↩︎
- https://www.biorxiv.org/content/10.1101/643767v2 ↩︎
- https://arxiv.org/pdf/1902.07669.pdf ↩︎
- https://www.biorxiv.org/content/10.1101/643767v2.full.pdf ↩︎
- https://arxiv.org/pdf/2004.03497.pdf ↩︎
- https://www.nature.com/articles/s41586-021-03819-2 ↩︎
- https://www.nature.com/articles/s41586-021-03819-2 ↩︎
- https://en.wikipedia.org/wiki/CD4 ↩︎